This website uses cookies so that we can provide you with the best user experience possible. Cookie information is stored in your browser and performs functions such as recognising you when you return to our website and helping our team to understand which sections of the website you find most interesting and useful.
OPINION ARTICLE: Data Quality – the steppingstone to Sustainable Mobility
Tu-Tho Thai is a manager for projects and partnerships at ITxPT, a non-profit association enabling interoperability between IT systems in Public Transport. As an expert of data standards and related services, she discussed in the framework of MobiDataLab the challenges and importance of quality in data sharing for the transport sector.
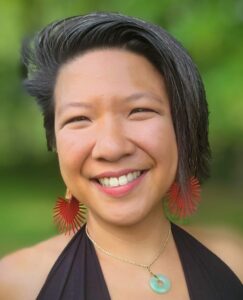
Looking at the different challenges presented by cities via POLIS for the MobiDataLab Living Labs, I was particularly interested in Milan’s proposal to work on data quality. As a topic that I have been very vocal about for the past years, I was very pleased to see how much it also resonated with the teams, though it underlined how much needed better focus on data quality is.
Data for the sake of data is a waste of resources
First, let’s be honest: data has become the “hot” topic of mobility conferences for the past five years or more. At every single panel, keynote, or workshop, all you will hear is “data”. However, if you listen closely to all the speakers, you will soon realise two things:
- All of them refer to different types of data, which will be specific to their field of expertise (e.g., floating car data, travellers’ information, transactional data, etc.),
- Most of them will show you all the things they can do with data while omitting what it takes for their solutions to work.
Though they are not wrong in stating that we need data to make better informed decision, they omit that data comes at a very high cost. A lot of resources are required to:
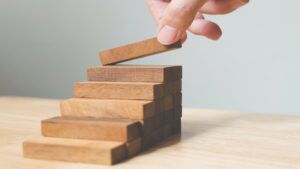
- Conceive, produce, and install the right hardware to generate raw data,
- Collect and consolidate the raw data to publish it into the suitable format,
- Share the consolidated data (hereinafter referred to as dataset) with the interested parties,
- Integrate the dataset into a dashboard or wider-scale information that other parties can act upon.
The last of the abovementioned steps is the crucial one: if the dataset cannot be integrated or consumed directly or easily, the resources spent on generating data are a waste of resources. Like producing clothes that will never get worn, it fuels climate change.
To avoid this, there are two major steps to be taken before data is even produced or collected:
- Define what are the questions you want to be answered with data – as trivial as it can sound, it is essential to prevent data flooding or investing in technology that is not required,
- Make sure that the data you will handle is standardised using existing mobility standards (e.g., SIRI, DATEX-II) or widely spread technical specifications (e.g., GTFS Schedule, MDS) to ensure data portability and interoperability.
Investing in Data Quality
Yet, standardisation is not enough to ensure that the dataset can be consumed by a third-party application or integrated into an analytic algorithm. Similarly, submitting a blank pdf file for an exam to comply with the teacher’s request is pointless to get a passing grade.
To be used, any dataset must be of a certain quality. Here, quality is defined based on the dataset:
- Relevance – does the dataset help answer the question (e.g., can the list of docking stations for bikes be used to define new mobility hubs?),
- Consistency – is the dataset in the correct/expected format (e.g., is the NeTEx dataset expressed using .xml files?),
- Accuracy – does the dataset have entries that are correctly entered (e.g., does it have the correct number of cars available in the city’s carsharing scheme?),
- Completeness – does the dataset represent all the elements we are supposed to take into consideration (e.g., does it represent all the lines of a public transport system?),
- Timeliness – is the latest dataset still valid (e.g., is the dataset produced 6 months ago still valid and can it be used to make decisions?).
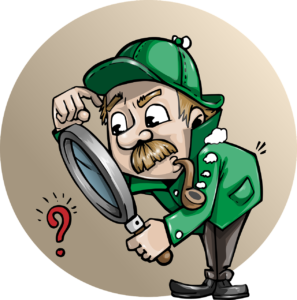
The criterion of relevance is tied into defining the questions we want answered with the data. The criterion of consistency is the “easiest” one to address: investigate the existing validators, often open-source and free to use, that are developed by the same team working on standards (e.g., NeTEx canonical validator by DATA4PT). Rely on what the community has done and include them in the data pipeline. Invest by giving feedback, time, or resources for them to keep on doing their work.
It is for the three other criteria left that investment is much needed in two folds:
- A change in mindset to make sure that producing a dataset is not the end-goal of your workflow, as quality is a perpetual improvement with datasets that must be maintained and meaningful for others to use them,
- An investment in tools and resources to build capacity and to integrate data production, consolidation, and improvement in your workflow.
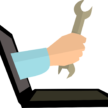
The investment starts now by looking at the solutions issued from the MobiDataLab Living Labs, and by exploring what other organisations or ecosystems have built. Data quality must be a systemic approach.
Follow @MobiDataLab on Twitter and Linkedin for more mobility data content!
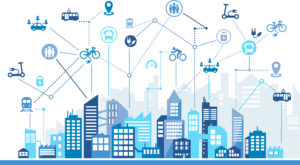